Adapting to the Unknown: Embracing the Evolution of Cybersecurity Models in the Digital Era
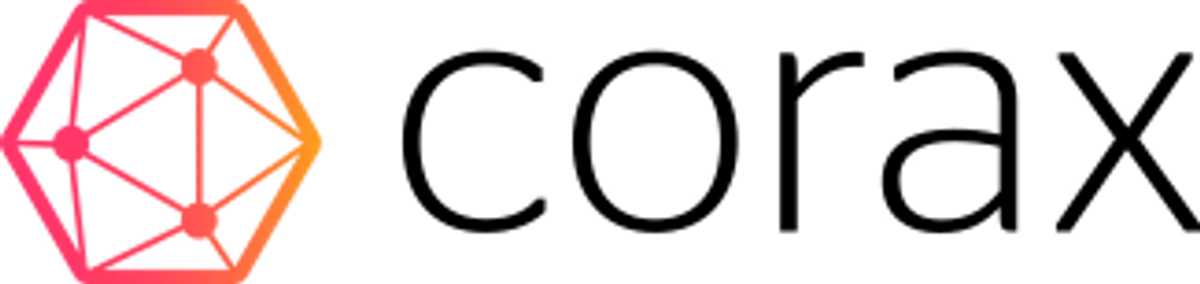
Introduction
In today’s highly interconnected world, the threat landscape for cybersecurity is constantly evolving. Cyber-attacks have become increasingly sophisticated and frequent, posing significant challenges for organizations of all sizes and industries. In response to these new and evolving threats, the cybersecurity community recognizes the need to develop and adopt new models of cybersecurity assessment. These models utilize data-driven approaches, probabilistic methods, and AI-assisted predictive modeling to better assess, quantify, and mitigate various cybersecurity threats. This article explores the importance of adapting to the unknown and embracing the evolution of cybersecurity models in the digital era.
Understanding the Limitations of Traditional Approaches
Conventional cybersecurity assessment methods typically rely on deterministic models that struggle to keep pace with the constantly changing and evolving nature of cyber threats. These approaches are founded on predefined rules and signatures, rendering them less effective at detecting and mitigating emerging threats. Moreover, deterministic models may overlook the uncertainties and complexities inherent in the cyber threat landscape, leading to gaps in cybersecurity defenses.
The Shift Towards Data-Driven Models
There is a growing need to utilize data-driven models for evaluating cybersecurity, which can address the limitations of traditional approaches. By analyzing large volumes of varied data sources, such as network logs, threat intelligence feeds, and security incident reports, organizations can gain critical insights into emerging threats and vulnerabilities. Employing big data analytics and machine learning techniques enables organizations to identify patterns, trends, and anomalies that signal cyber-attacks. This empowers them to take proactive steps to protect their digital assets.
Embracing Probabilistic Models
Probabilistic modeling provides a more adaptive and flexible approach to cybersecurity assessment by allowing organizations to account for uncertainties and variability in the cyber threat landscape. Unlike deterministic models, which depend on predefined rules and thresholds, probabilistic models evaluate the likelihood of various outcomes and assign probabilities to different scenarios. This empowers organizations to make more informed decisions about cybersecurity risk management and allocate resources more effectively to mitigate the most significant threats.
AI-Assisted Predictive Models
Artificial intelligence plays a crucial role in enhancing the predictive capabilities of cybersecurity models. By leveraging AI algorithms, organizations can analyze vast amounts of data in real-time and identify potential cyber threats before they materialize. AI-assisted predictive models enable organizations to detect anomalies and recognize patterns indicative of malicious activity, allowing them to respond to cyber-attacks more effectively. This proactive approach to cybersecurity empowers organizations to stay one step ahead of cyber adversaries and mitigate the risk of costly data breaches and cyber-attacks.
Challenges and Considerations
While data-driven, probabilistic, and AI-assisted cybersecurity models offer significant benefits, they also present various challenges. Organizations must address ethical and privacy concerns related to the collection and analysis of large volumes of sensitive data. Additionally, technical challenges exist in implementing AI-assisted predictive models, including data integration, model training, and algorithm selection. Ensuring transparency and accountability in decision-making processes is crucial for building trust and confidence in these advanced cybersecurity models.
Benefits and Opportunities
The adoption of data-driven, probabilistic, and AI-assisted cybersecurity models provides numerous advantages and opportunities for organizations. These models help organizations enhance the accuracy and effectiveness of cyber threat detection and mitigation, improve adaptability to dynamic and evolving threats, and support proactive decision-making in cybersecurity risk management. By embracing the evolution of cybersecurity models in the digital era, organizations can bolster their cybersecurity posture and safeguard their digital assets against emerging cyber threats.
Many organizations in various industries are already enjoying the advantages of data-driven, probabilistic, and AI-assisted cybersecurity models. Real-world examples showcase the efficiency of these models in identifying and addressing cyber threats, minimizing the risk of data breaches, and protecting critical infrastructure and information systems.
Conclusion
In conclusion, the evolution of cybersecurity models in the digital era is essential for organizations to effectively address the dynamic and evolving nature of cyber threats. By adopting data-driven, probabilistic, and AI-assisted approaches to cybersecurity assessment, organizations can improve their ability to detect, quantify, and mitigate cyber risks. It is time to adapt to the unknown and embrace the evolution of cybersecurity models to protect against emerging cyber threats and safeguard digital assets in an increasingly interconnected world.